At the beginning of 2024, the sauce-flavored liquor company Sijiu Fang launched a large model-driven chatbot in their dealer group chat.
Chat group bots are not new, but large models are a trendy technology. Sijiu Fang is a newcomer in the liquor industry, established only in 2015. Compared to traditional liquor companies, it is not a large enterprise, but it is sensitive to new technologies. The CIO (Chief Information Officer) of Sijiu Fang, Zhang Peng, told us that dealers are too important for the liquor company, and he hopes to improve service capabilities for dealers by leveraging the natural language interaction functions of large models, with the aim of increasing sales.
After a period of excitement, Zhang Peng was a bit disappointed, "The large model-empowered robot is indeed different, it can talk a lot, and it can provide emotional value even more than a close friend, but this is not business value."
There are mainly two ways for large models to be implemented in enterprises. One is for the enterprise to privately deploy a large model, which can ensure the security of the enterprise's data, but the deployment cost is as high as several million yuan, suitable for data-sensitive industries such as finance, telecommunications, and energy. The other is to call the manufacturer's large model API (Application Programming Interface), which has a low cost, mainly billed based on the quantity of Tokens (the smallest unit that the model can understand and generate, which can be a word, a number, or a punctuation mark, etc.), and the deployment method is simple, suitable for a wider range of scenarios.
Advertisement
Sijiu Fang mainly adopts the low-cost API call mode. After the pilot, the company found that the large model has limited business value, and decision-makers have become more conservative about the subsequent exploration of large model implementation.
We communicated with more than ten CIOs from leading companies in various industries (mainly relatively traditional industries) of different sizes about the implementation of large models, and received two feedbacks:
Firstly, as the most important innovative technology currently considered, large models are indeed valued by enterprises. They want to solve the problems of pain point business scenarios through large model technology, bringing practical value such as cost reduction, efficiency increase, quality improvement, demand pulling, and profit creation to the enterprise.
Secondly, the current large model technology is mainly implemented in single points and small scenarios, and it is difficult to bring direct returns to the overall business. The Chief Technology Officer of a leading coffee chain brand said that large models can only add icing on the cake, not provide timely help.
At present, the main obstacles for most enterprises to implement large models include but are not limited to the following four points: the limited number of landing scenarios with clear commercial value, insufficient engineering capabilities for model implementation, imperfect large models in the industry and scenarios, and the enterprise's own insufficient understanding of large models.The meta-view data provides collateral support for the above feedback. Guolian Securities has checked the financial reports of all A-share companies for the year 2023, and 883 listed companies mentioned generative AI business. The companies that have implemented this are concentrated in the TMT (Technology, Media, Telecom) industry and large-cap companies. More than half of the primary industry penetration rates are less than 10%, and the overall penetration rate of A-share listed companies is less than 20%.
From the results, the scenarios explored by pioneering companies are limited, focusing on virtual humans, customer service Q&A, marketing copywriting, graphic design, code generation, knowledge bases, and intelligent assistants. These scenarios have a relatively light connection with the core business of the companies. Since the content generated by large models is closely related to the quality of training data and prompt words, the implementation effects of typical scenarios are also quite uneven.
Most of the companies that have adopted large model technology are those that are sensitive to technology and have a relatively solid digital foundation. Other companies still need to catch up in these two areas to implement large models. Across industries, there is a lot of discussion about large models but less implementation. The deputy general manager of a leading company in the pharmaceutical distribution industry told us that, in his view, 2024 is not the year when large models will be implemented in his industry: there is a lack of talent, awareness, and technology.
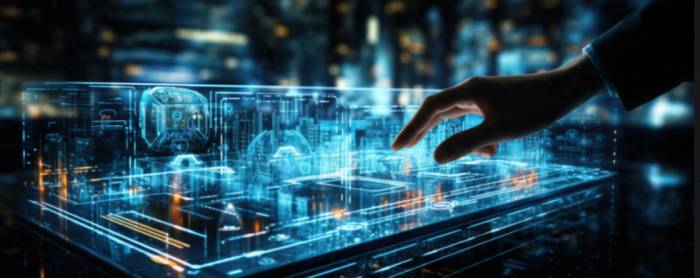
This means that currently, large models are being implemented in industries that are naturally close to digitalization, while more traditional industries are still in a wait-and-see state. This is in line with the law of new technology promotion and also poses a question for technology companies of the times—if large models are indeed a major technology that can promote industrial innovation, how should their implementation path be in a broader range of industries?
"I won't pay for toys."
The steps for companies to implement large models are generally divided into three steps:
First, the IT team sorts out the performance characteristics of open and closed-source models on the market. Different models have obvious differences; some have strong semantic understanding capabilities but are not sensitive to data and time; some have strong logical reasoning but poor language expression; and some are strong in English scenarios but weak in Chinese scenarios...
Second, identify the pain points of each business line, that is, the intersection of large model technology and business needs.
Third, assess the specific scenarios suitable for large models.
For this, the technical team needs to formulate a technical budget, invest in human resources, and coordinate cross-departmental cooperation. However, even so, the business after the implementation of large models may not necessarily gain more commercial value.The person in charge of Smart Spectrum AI told us that after more than a year of development in the large model industry, domestic enterprise customers have shifted their focus from parameters and rankings in 2023 to value transformation in 2024. This means that the demand on the enterprise side has undergone a transformation from "model is king" to "commercial value is king." This requires companies to find the greatest common divisor of large model capabilities, enterprise scenarios, and business nodes.
This is not an easy task. Zhang Peng analyzed that the chatbot in his dealer group is a marketing tool. After being upgraded with large model technology, the language expression of the chatbot has become more natural, but it still lacks the proactive service and professional quality of sales personnel. Its core function is to synchronize product prices, channels, and other information or industry general knowledge. Although the overall capability has improved compared to the previous generation of Q&A robots, the business value is not obvious.
At first, Zhang Peng chose to implement large model technology with chatbots because the marketing scenario can directly evaluate the transformation of commercial value. However, from the results, the input and output of this scenario "do not quite meet expectations."
The CTO of the aforementioned leading coffee chain brand believes that large model technology has demonstrated some value as an efficiency tool in business. The company has introduced large models into marketing, customer service, and internal training scenarios, where large models "have some effect" in assisting with copywriting and design creativity. However, he evaluates that efficiency value is relative, and business value is more important for companies. Therefore, his priority for the subsequent implementation of large models will not be high.
Several CIOs of industrial enterprises told us that they hope model manufacturers will provide more benchmark cases for the implementation of large models. However, the main focus of domestic leading manufacturers is on breaking through the underlying large models to catch up with overseas companies. They generally maintain a rhythm of iterating the underlying large models every three months. Therefore, when most model manufacturers communicate with companies, they mainly promote technology rather than provide industry solutions.
A common misunderstanding in the implementation of large models in enterprises is to focus on the capabilities of large models first, looking for nails with a hammer. But the reality is that not all applications can generate commercial value by redoing them with large models now.
Haidilao began to get in touch with large model technology at the beginning of 2023. Like most companies, they followed the general impression of large models at the time and introduced them into scenarios such as automatic generation of marketing copy and Feishu Q&A robots. Yang Xuanzhi, the product person in charge of Haidilao's information technology department, summarized that the applications at that stage were "more like toys, more experimental," and did not help the business much.
To find value scenarios, companies often need a period of exploration. It was not until the beginning of 2024 that Haidilao made a breakthrough. Yang Xuanzhi summarized that large models are suitable for combining with mature small models to implement scenarios of repetitive labor.
A typical scenario is that at the beginning of 2024, Haidilao introduced large model technology into the material identification process of the central warehouse. The central warehouse is the distribution center for goods in Haidilao stores, receiving products from all over the world. There is a big difference in the language and printing and packaging of the goods. In the past, the central warehouse used OCR (Optical Character Recognition technology, which is a technology that scans and recognizes text information in images and converts it into an editable, searchable text format) technology to identify and extract information such as the production date, shelf life, and manufacturer of the materials, and then manually classified the information, organizing the material information into a system-recognizable format document for use by upstream and downstream units of the group company.
Yang Xuanzhi explained that OCR is a small model that solves the problem of information recognition and extraction, and the subsequent information classification is a manual repetitive labor scenario. Manual information classification has problems such as non-uniform translation standards, long time consumption, and high costs. Previously, the cost of classifying a piece of Chinese material information in Haidilao's central warehouse was about 50 cents, and classifying a piece of foreign material information was 80 cents. Now, with the introduction of the large model's natural language understanding capability, this work can be completed by the system at a high cost-performance ratio.Few companies can afford to spend a year trying out large models in various scenarios, akin to the approach taken by Haidilao. The majority of private enterprises have reduced their innovation budgets amid economic headwinds. If a business scenario is incorrectly chosen early on in the deployment of large models, the company's exploration will be superficial and quickly abandoned.
Since May 2024, domestic large model manufacturers have collectively announced a reduction in the cost of accessing model APIs, aiming to lower the cost of enterprise exploration of large models and attract more companies to join the innovative ecosystem of large model applications.
A core member from a leading domestic large model manufacturer commented that the price reduction is not to wage a price war, but to attract customers. Technology companies that have adopted large models have actually taken a step ahead. From the perspective of other industries, many enterprises have not yet reached the stage of paying for large models.
New weapons, hard to master
In the world of martial arts, the unity of man and sword symbolizes the highest realm of martial arts. In the wave of large models, only when large models are integrated into a company's business and development can they exert the imagined power. This means that the company's systems and data must be fully integrated with the large model. This is an extreme test of the company's digital construction and engineering development capabilities, and companies with insufficient foundations may not even be able to handle this new weapon.
Specifically, there are two prerequisites for the core business of a company to implement large models. First, the company has built a digital system, and multiple systems can be interconnected to achieve interoperability. Second, the company has achieved the integration and governance of internal data.
The former tests the company's past level of digital construction. Only companies that have interconnected internal management tools and productivity tools such as OA (Office Automation System), ERP (Enterprise Resource Planning System), MES (Manufacturing Execution System), CRM (Customer Relationship Management System), etc., can provide convenience for the implementation of large models.
The latter requires companies to mine massive amounts of structured data in various formats such as Excel, CSV, XML, and unstructured data such as audio, video, and images scattered everywhere, and complete data cleaning, preprocessing, tagging, and vectorization processing. Only then can large models understand and use the company's data.
Traditionally, the IT departments in industrial enterprises have mainly taken on the role of procurement, selecting and purchasing appropriate technical solutions for business scenarios, and rarely undertake specific development work. IT departments can spend a lot of money to purchase external services to complete the construction of corporate digital systems. However, large models have also put forward new requirements for companies—how to combine the original digital foundation of the company with large models, and how to transform professionals' understanding of business scenarios into knowledge bases and knowledge governance, all of which require a lot of engineering work.
At present, the implementation of large models is faster in companies that have invested a lot of time and money in intelligentization. Their digital foundation is more complete, and model manufacturers are also willing to provide tailored on-site services for these few leading companies.However, the vast majority of industries consist of businesses that are not as large-scale and have limited annual investments in digitalization. Their digital construction and engineering development capabilities lag behind, and to make good use of large models, they require more tailored engineering customization services. Large model vendors, due to reasons such as cost control and limited resources, are unable to meet these needs.
This is a pain point for the current implementation of large models and also an opportunity for the future—large model implementation requires a group of third-party development service providers.
The head of digitalization at a leading consumer goods company told us that many software companies in the United States are exploring engineering services for the implementation of large model scenarios, but there is still a gap in this ecosystem in China. Existing service providers focus on the private deployment of large models, while there is insufficient attention to the in-depth use of large model API calls. At the same time, regardless of the implementation method, the engineering solutions that combine large models with corporate value scenarios are immature.
The lack of relevant solutions will only allow enterprises to integrate large models into single business nodes, rather than implementing large model applications in more complex business scenarios.
Mengniu's progress in implementing large models is in the first tier among domestic companies. Its digital intelligence team began to explore and implement large model technology in the second half of 2022. Mengniu's Chief Digital Intelligence Officer, Li Chengjie, told us that the company has accumulated a set of methodologies for the implementation of large models internally.
Mengniu has built an "1+2" AI technology infrastructure. The "1" is the AI infrastructure, which can efficiently and performantly schedule multiple models while ensuring service compliance and data security. The "2" consists of the corporate brain and the knowledge bank. The corporate brain is responsible for connecting and scheduling large and small models, accessing and calling existing capabilities of the business middleware through API methods, and achieving the integration of internal corporate systems. The knowledge bank, after fully mining corporate data, processes the data into a quantifiable form, making it AI-friendly knowledge, and accumulates and governs Mengniu's industry knowledge.
Above the technology infrastructure is the AI Agent Builder low-code platform, which allows business personnel to quickly build AI Agents (software entities with a certain level of intelligence that can complete specific tasks autonomously or under user guidance) through drag-and-drop methods to empower specific business scenarios. Li Chengjie told us that if an enterprise can only use large models at a single node, the value it can produce is not significant. Taking the marketing scenario as an example, Mengniu has achieved end-to-end process optimization assisted by large models in marketing deployment decisions and execution.
In terms of content strategy, large models will combine social media hotspots, brand and product information to automatically generate creative concepts and content frameworks. The generation of specific marketing content takes into account factors such as communication platform positioning, account positioning, and keyword strategies. In terms of audience decision-making, large models automatically recommend based on deployment targets, budgets, media attributes, and target audiences. In terms of deployment effect analysis, large models generate effect assessments based on industry and Mengniu's past experiences, public sentiment, and deployment targets.
The entire process mobilizes the joint efforts of knowledge management Agents, data scheduling Agents, and information analysis Agents. Now, Mengniu's marketing deployment response rate has increased by 12% compared to manual methods.
Li Chengjie summarized Mengniu's experience in implementing large models: only with the right AI infrastructure + deployable AI components + company-specific knowledge/process customization can enterprises achieve end-to-end process optimization and efficiency improvement.In the past, Independent Software Vendors (ISVs) provided general solutions across different fields to meet the specific needs of enterprises. However, the application of large models is based on the understanding of business scenarios, knowledge sorting, knowledge enhancement, knowledge distribution, and application. Few ISVs have mastered the knowledge of enterprise scenarios, leaving a lot of gaps in the market.
Many manufacturers have started to respond to customer needs by providing underlying engineering capabilities for model deployment. However, due to their unfamiliarity with business scenarios, their products are difficult to meet the actual needs of enterprises, and "good ones are as rare as phoenix feathers and qilin horns."
At this stage, the service ecosystem for the landing of domestic models is not yet mature, and the landing of large models depends on the coordination and development capabilities of the enterprise's technical team. This has invisibly raised the threshold for the landing of large models, and also makes it difficult to develop more innovative application scenarios based on general large models.
Industry and scenario models are not yet perfect.
The lack of landing service providers leads to the inability of general large models to fully release their capabilities in business scenarios. In industries and vertical scenarios where the capabilities of general large models are not covered, models that are more suitable are also in a state of absence.
At present, the main force of commercialization of major manufacturers is general large models. Its knowledge base is broad but not deep enough, and its practicality in the industry is insufficient. In contrast, industry and scenario models are experts, focusing on specific scenario needs, and can provide more business value.
On the one hand, industries with high professional barriers such as construction, chemical, and medical health need industry models. Taking the construction industry as an example, construction projects are usually large in scale, involving design, construction, management, and other stages, and need to process a large amount of data and information. However, the construction industry has unique professional knowledge and standard specifications, and the processing of related data and information requires the model to understand and apply professional knowledge, which general large models are usually difficult to cope with the needs of professional construction projects.
Since the second half of last year, many manufacturers have launched industry large models. However, the consensus in the industry is that the capabilities of these industry large models are extremely limited.
Many CIOs of industrial enterprises believe that there is a paradox in asking model manufacturers to provide industry models. The data used by model manufacturers to train large models generally comes from publicly available data on the Internet, purchased external data, and enterprise-owned data. These data are mainly general and difficult to cover specific industries. Industry data is mainly in the hands of the leading enterprises in the industry. However, most industry enterprises do not have the R&D strength of model manufacturers, and it is also difficult to develop industry large models without leaving the main business.
In response to this, the mainstream approach of model manufacturers is to co-develop industry large models with leading enterprises in the industry. However, model manufacturers cannot take the enterprise's data as their own, which cannot solve the problem of the source of industry data.A perspective suggests that leading companies in an industry, after deploying privatized large models, can export their experiences as industry solutions. Another viewpoint posits that technology companies in niche fields also have the opportunity to become drivers of industry-wide large models. However, several digitalization heads from leading companies in consumer, supply chain, and catering industries have indicated that they have not seen a mature privatized model within their sectors.
The consequence of lacking industry-specific models is that the accuracy of large models is insufficient, and their compatibility with the core business of the industry is low. A representative from an agency company that provides privatized model deployment for top domestic manufacturers told us that, to date, he has pitched large models to 4-5 companies in the energy, finance, and telecommunications industries. Initially, these companies were interested, and two of them even reached the POC (Proof of Concepts) stage, which is a solution to verify the effectiveness and applicability of a concept, but none of them ultimately made a purchase.
The core reason is the insufficient accuracy of large models, which can reach a maximum of around 70%. For enterprise-level applications, the higher the accuracy of large models, the better, with a threshold of 95%. The main solution from domestic model manufacturers currently is to improve the underlying model capabilities to enhance the accuracy of large model applications.
On the other hand, the absence of specific scenario models also hinders the implementation of large models. Sìshí Jiǔ Fāng is exploring the application of image models in liquor packaging design. They have tried multiple open-source and closed-source generative image models but have not found any products that can be directly applied.
Zhang Peng explained that packaging design is different from other graphic designs, requiring images with a resolution of over 10M for printing; the core target audience for the company's products is middle-aged men in China, and the packaging design must cater to their aesthetic preferences; the packaging design must also include trademark text information.
Currently, the image precision generated by text-to-image models on the market does not meet printing requirements; the image style is mainly anime, and even with minor adjustments to the model, it is difficult to generate satisfactory images that fit the Chinese and new Chinese styles of sauce liquor design; there are also limited models that can accurately generate text on images.
In theory, service providers could retrain image models and develop them into engineering applications to create a large model application for packaging design. However, the few service providers that Zhang Peng has contacted have shown little interest in this. This is because it implies an investment of hundreds of thousands of dollars in research and development and a longer commercial transformation cycle.
Most service providers only wish to sell generic large model technology and bundle it with ready-made supporting facilities such as computing power and cloud services. Brand owners lack the capability to train models to solve industry-specific problems. This creates a significant gap in the demand for services.
Large model talent is both expensive and scarce.
It is a fact that only a minority of companies can currently implement large model technology. Most companies, due to weak digital foundations, lack the talent and accumulated knowledge of large model technology.The digitalization head of a top global beverage's domestic distributor told us that his company has been posting recruitment notices through various channels since January of this year, hoping to find a large model technology talent with a consulting background to promote the implementation of technology. By late May, this person was still not found, and the technical solutions provided by his department were still traditional decision-making AI.
Traditional enterprises that cannot cultivate AI talent internally or introduce it from the outside will find it difficult to bridge the cognitive gap in new technologies.
For industrial enterprises, it is very difficult to introduce large model talents from the outside because they have to compete with technology companies. The "2023 AI Talent Insight" released by Maimai GaoPeng Talent Think Tank in November 2023 shows that internet, new lifestyle services, and gaming companies have the greatest demand for AI talent. The average salary offered for new AI positions in the first eight months of 2023 was 46,518 yuan, an increase of 6.16% compared to 2022.
Among them, data mining, algorithm researchers, and algorithm engineers have an average monthly salary of more than 51,000 yuan, and an annual salary (excluding year-end bonus) of more than 610,000 yuan. In contrast, the National Bureau of Statistics announced in 2023 that the average annual salary for IT positions (urban private units in the information transmission, software, and information technology service industry) serving social production and life services was only 156,000 yuan.
AI talent can bring technological cognition and reserves. Companies that are willing to recruit AI talent and already have AI talent reserves are more likely to implement large models. Due to the high cost of AI talent investment, the attitude of corporate decision-makers towards introducing AI talent and implementing large models will play a key role.
Yang Xuanzhi pointed out that decision-makers who have benefited from digital construction in the past are more willing to invest in new technologies. Otherwise, even if professional technical personnel tell them the value of large models, decision-makers will find it difficult to understand and believe. "Only those who have seen, used, and experienced the benefits of technology are willing to invest when new technologies emerge."
Mengniu and Haidilao's rapid implementation of large models is because the company's decision-makers have already tasted the benefits of digitalization. Haidilao has increased its investment in digital transformation since 2016. The company's financial report shows that 20% of the proceeds from Haidilao's listing in 2018 (about 1.46 billion Hong Kong dollars) will be used for "development and use of new technologies," and since 2018, this fund has been consumed by tens of millions of Hong Kong dollars every year.
Yang Xuanzhi mentioned that Haidilao's corporate culture and organizational structure support the technical team in testing large models in multiple business scenarios. At the same time, the catering industry where Haidilao is located has developed for thousands of years, with strong industry commonality, which is suitable for the current implementation of large models. As a leading company in the industry, Haidilao can obtain personalized services from model manufacturers to solve the engineering problems of large model implementation.
However, companies like Mengniu and Haidilao that have invested in innovative technologies and benefited in the past few years are a minority. The "2023 China Enterprise Digital Transformation Index" released by Accenture, an international management consulting and information technology services company, shows that affected by the macro environment, Chinese companies focus on operations and cost optimization, and the intensity of innovation has significantly weakened. The proportion of active businesses in innovation in 2023 has dropped to 9%, about half of that in 2022. In the past six years, the peak value of surveyed companies participating in digital business innovation accounted for 17% of the total.The good news is that the perception of large model technology by enterprises is not static. It is closely related to the commercial value of large model applications, benchmark cases, and the implementation of industry models.
The deputy general manager of a leading company in the pharmaceutical distribution industry told us that, influenced by relevant favorable factors, even companies that have not yet established an AI team may quickly adopt large model technology to gain benefits. Especially the leading companies in traditional industries, they have the advantage of being latecomers. When the value of large models to the industry becomes apparent, they can acquire relevant capabilities through investment and mergers and acquisitions.
Since 2023, AI large models have become the new engine of the technology industry, attracting a large amount of capital and giving birth to several unicorn companies. However, whether large models are a temporary bubble or a tool with substantial business value, this question has been lingering in the minds of many corporate decision-makers. Several interviewed CIOs have indicated that this year is the first year for large models to explore and implement in some industries. The value of large models will be answered in the practice of implementation.
POST A COMMENT